Causal Reinforcement Learning
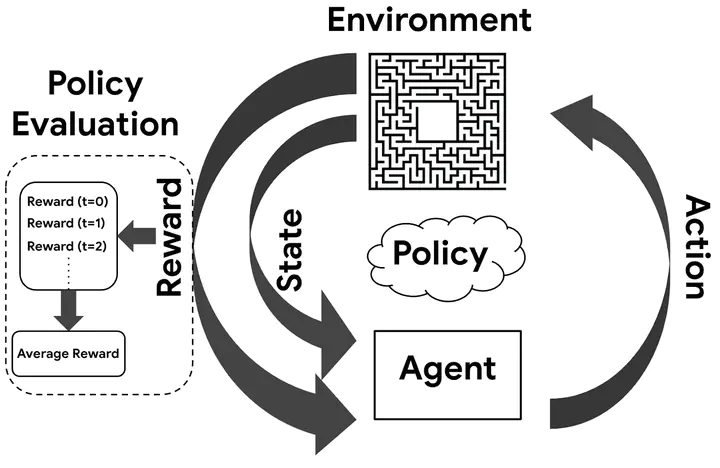
In the standard data analysis framework, data is first collected (once for all), and then data analysis is carried out. Moreover, the data-generating process is typically assumed to be exogenous. This approach is natural when the data analyst has no impact on how the data is generated. The advancement of digital technology, however, has facilitated firms to learn from data and make decisions at the same time. As these decisions generate new data, the data analyst—a business manager or an algorithm—also becomes the data generator. This interaction generates a new type of bias—reinforcement bias—that exacerbates the endogeneity problem in static data analysis. Causal inference techniques ought to be incorporated into reinforcement learning to address such issues.